mirror of
https://github.com/clearml/clearml
synced 2025-05-01 11:26:43 +00:00
Documentation
This commit is contained in:
parent
22b18e7338
commit
a0ecc6d516
149
README.md
149
README.md
@ -1,127 +1,72 @@
|
||||
# TRAINS
|
||||
## Auto-Magical Experiment Manager & Version Control for AI
|
||||
|
||||
<p style="font-size:1.2rem; font-weight:700;">"Because it’s a jungle out there"</p>
|
||||
"Because it’s a jungle out there"
|
||||
|
||||
[](https://img.shields.io/github/license/allegroai/trains.svg)
|
||||
[](https://img.shields.io/pypi/pyversions/trains.svg)
|
||||
[](https://img.shields.io/pypi/v/trains.svg)
|
||||
[](https://pypi.python.org/pypi/trains/)
|
||||
|
||||
Behind every great scientist are great repeatable methods. Sadly, this is easier said than done.
|
||||
|
||||
When talented scientists, engineers, or developers work on their own, a mess may be unavoidable.
|
||||
Yet, it may still be manageable. However, with time and more people joining your project, managing the clutter takes
|
||||
its toll on productivity. As your project moves toward production, visibility and provenance for scaling your
|
||||
deep-learning efforts are a must.
|
||||
|
||||
For teams or entire companies, TRAINS logs everything in one central server and takes on the responsibilities for
|
||||
visibility and provenance so productivity does not suffer. TRAINS records and manages various deep learning
|
||||
research workloads and does so with practically zero integration costs.
|
||||
TRAINS is our solution to a problem we share with countless other researchers and developers in the machine
|
||||
learning/deep learning universe: Training production-grade deep learning models is a glorious but messy process.
|
||||
TRAINS tracks and controls the process by associating code version control, research projects,
|
||||
performance metrics, and model provenance.
|
||||
|
||||
We designed TRAINS specifically to require effortless integration so that teams can preserve their existing methods
|
||||
and practices. Use it on a daily basis to boost collaboration and visibility, or use it to automatically collect
|
||||
your experimentation logs, outputs, and data to one centralized server.
|
||||
|
||||
(See TRAINS live at [https://demoapp.trainsai.io](https://demoapp.trainsai.io))
|
||||
(Experience TRAINS live at [https://demoapp.trainsai.io](https://demoapp.trainsai.io))
|
||||
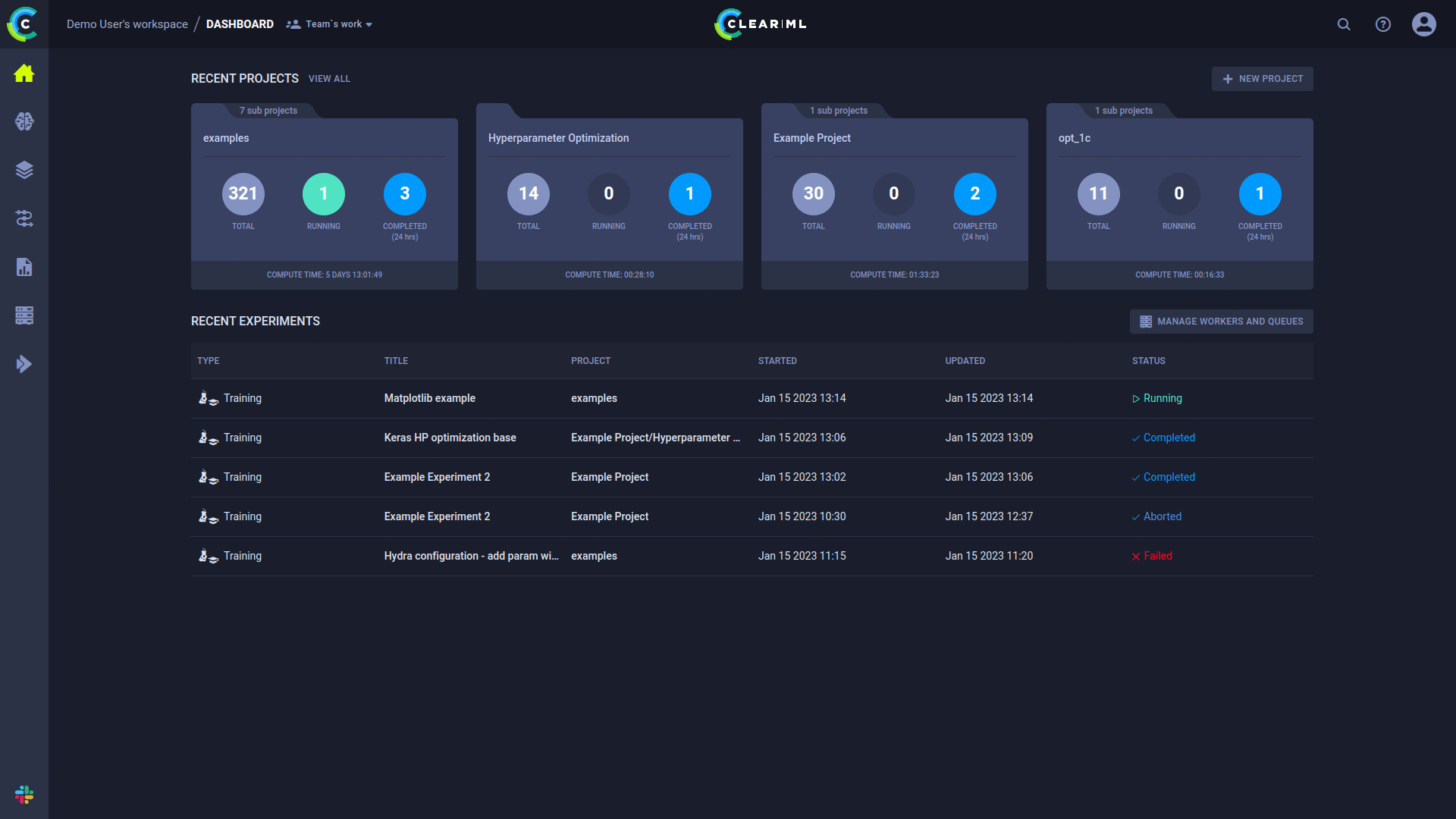
|
||||
|
||||
|
||||
## Main Features
|
||||
|
||||
TRAINS is our solution to a problem we shared with countless other researchers and developers in the machine
|
||||
learning/deep learning universe: Training production-grade deep learning models is a glorious but messy process.
|
||||
TRAINS tracks and controls the process by associating code version control, research projects,
|
||||
performance metrics, and model provenance.
|
||||
|
||||
* Start today!
|
||||
* TRAINS is free and open-source
|
||||
* TRAINS requires only two lines of code for full integration
|
||||
* Use it with your favorite tools
|
||||
* Seamless integration with leading frameworks, including: *PyTorch*, *TensorFlow*, *Keras*, and others coming soon
|
||||
* Support for *Jupyter Notebook* (see [trains-jupyter-plugin](https://github.com/allegroai/trains-jupyter-plugin))
|
||||
and *PyCharm* remote debugging (see [trains-pycharm-plugin](https://github.com/allegroai/trains-pycharm-plugin))
|
||||
* Log everything. Experiments become truly repeatable
|
||||
* Model logging with **automatic association** of **model + code + parameters + initial weights**
|
||||
* Automatically create a copy of models on centralized storage
|
||||
([supports shared folders, S3, GS,](https://github.com/allegroai/trains/blob/master/docs/faq.md#i-read-there-is-a-feature-for-centralized-model-storage-how-do-i-use-it-) and Azure is coming soon!)
|
||||
* Seamless integration with leading frameworks, including: *PyTorch*, *TensorFlow*, *Keras*, and others coming soon
|
||||
* Support for *Jupyter Notebook* and *PyCharm* remote debugging
|
||||
* Automatic log collection.
|
||||
* Query, Filter, and Compare your experiment data and results
|
||||
* Share and collaborate
|
||||
* Multi-user process tracking and collaboration
|
||||
* Centralized server for aggregating logs, records, and general bookkeeping
|
||||
* Increase productivity
|
||||
* Comprehensive **experiment comparison**: code commits, initial weights, hyper-parameters and metric results
|
||||
* Order & Organization
|
||||
* Manage and organize your experiments in projects
|
||||
* Query capabilities; sort and filter experiments by results metrics
|
||||
* And more
|
||||
* Stop an experiment on a remote machine using the web-app
|
||||
* A field-tested, feature-rich SDK for your on-the-fly customization needs
|
||||
|
||||
**Detailed overview of TRAINS offering and system design can be found [Here](https://github.com/allegroai/trains/blob/master/docs/brief.md).**
|
||||
|
||||
|
||||
## TRAINS Automatically Logs
|
||||
|
||||
* Git repository, branch, commit id and entry point (git diff coming soon)
|
||||
* Hyper-parameters, including
|
||||
* ArgParser for command line parameters with currently used values
|
||||
* Tensorflow Defines (absl-py)
|
||||
* Explicit parameters dictionary
|
||||
* Initial model weights file
|
||||
* Model snapshots
|
||||
* stdout and stderr
|
||||
* Tensorboard/TensorboardX scalars, metrics, histograms, images (with audio coming soon)
|
||||
* Matplotlib
|
||||
|
||||
|
||||
## See for Yourself
|
||||
## Using TRAINS
|
||||
|
||||
We have a demo server up and running at https://demoapp.trainsai.io. You can try out TRAINS and test your code with it.
|
||||
Note that it resets every 24 hours and all of the data is deleted.
|
||||
|
||||
Connect your code with TRAINS:
|
||||
When you are ready to use your own TRAINS server, go ahead and [install *TRAINS-server*](#configuring-your-own-trains).
|
||||
|
||||
TRAINS requires only two lines of code for full integration.
|
||||
|
||||
To connect your code with TRAINS:
|
||||
|
||||
1. Install TRAINS
|
||||
|
||||
pip install trains
|
||||
|
||||
1. Add the following lines to your code
|
||||
2. Add the following lines to your code
|
||||
|
||||
from trains import Task
|
||||
task = Task.init(project_name="my project", task_name="my task")
|
||||
|
||||
1. Run your code. When TRAINS connects to the server, a link is printed. For example
|
||||
* If project_name is not provided, the repository name will be used instead
|
||||
* If task_name (experiment) is not provided, the current filename will be used instead
|
||||
|
||||
3. Run your code. When TRAINS connects to the server, a link is printed. For example
|
||||
|
||||
TRAINS Results page:
|
||||
https://demoapp.trainsai.io/projects/76e5e2d45e914f52880621fe64601e85/experiments/241f06ae0f5c4b27b8ce8b64890ce152/output/log
|
||||
|
||||
1. Open the link and view your experiment parameters, model and tensorboard metrics
|
||||
4. Open the link and view your experiment parameters, model and tensorboard metrics
|
||||
|
||||
## Configuring Your Own TRAINS
|
||||
|
||||
## How TRAINS Works
|
||||
1. Install and run *TRAINS-server* (see [Installing the TRAINS Server](https://github.com/allegroai/trains-server))
|
||||
|
||||
TRAINS is a two part solution:
|
||||
|
||||
1. TRAINS [python package](https://pypi.org/project/trains/) (auto-magically connects your code, see [Using TRAINS](#using-trains))
|
||||
2. [TRAINS-server](https://github.com/allegroai/trains-server) for logging, querying, control and UI ([Web-App](https://github.com/allegroai/trains-web))
|
||||
|
||||
The following diagram illustrates the interaction of the [TRAINS-server](https://github.com/allegroai/trains-server)
|
||||
and a GPU training machine using the TRAINS python package
|
||||
|
||||
<!---
|
||||

|
||||
-->
|
||||
<img src="https://github.com/allegroai/trains/blob/master/docs/system_diagram.png?raw=true" width="50%">
|
||||
|
||||
|
||||
## Installing and Configuring TRAINS
|
||||
|
||||
1. Install and run trains-server (see [Installing the TRAINS Server](https://github.com/allegroai/trains-server))
|
||||
|
||||
2. Install TRAINS package
|
||||
|
||||
pip install trains
|
||||
|
||||
3. Run the initial configuration wizard and follow the instructions to setup TRAINS package
|
||||
(http://**_trains-server ip_**:__port__ and user credentials)
|
||||
2. Run the initial configuration wizard for your TRAINS installation and follow the instructions to setup TRAINS package
|
||||
(http://**_trains-server-ip_**:__port__ and user credentials)
|
||||
|
||||
trains-init
|
||||
|
||||
@ -129,30 +74,10 @@ After installing and configuring, you can access your configuration file at `~/t
|
||||
|
||||
Sample configuration file available [here](https://github.com/allegroai/trains/blob/master/docs/trains.conf).
|
||||
|
||||
## Using TRAINS
|
||||
|
||||
Add the following two lines to the beginning of your code
|
||||
|
||||
from trains import Task
|
||||
task = Task.init(project_name, task_name)
|
||||
|
||||
* If project_name is not provided, the repository name will be used instead
|
||||
* If task_name (experiment) is not provided, the current filename will be used instead
|
||||
|
||||
Executing your script prints a direct link to the experiment results page, for example:
|
||||
|
||||
```bash
|
||||
TRAINS Results page:
|
||||
|
||||
https://demoapp.trainsai.io/projects/76e5e2d45e914f52880621fe64601e85/experiments/241f06ae0f5c4b27b8ce8b64890ce152/output/log
|
||||
```
|
||||
|
||||
*For more examples and use cases*, see [examples](https://github.com/allegroai/trains/blob/master/docs/trains_examples.md).
|
||||
|
||||

|
||||
|
||||
|
||||
## Who Supports TRAINS?
|
||||
## Who We Are
|
||||
|
||||
TRAINS is supported by the same team behind *allegro.ai*,
|
||||
where we build deep learning pipelines and infrastructure for enterprise companies.
|
||||
@ -172,13 +97,19 @@ even though this project is currently in the beta stage, your logs and data will
|
||||
|
||||
Apache License, Version 2.0 (see the [LICENSE](https://www.apache.org/licenses/LICENSE-2.0.html) for more information)
|
||||
|
||||
## Guidelines for Contributing
|
||||
## Community
|
||||
|
||||
If you have any questions, look to the TRAINS [FAQ](https://github.com/allegroai/trains/blob/master/docs/faq.md), or
|
||||
tag your questions on [stackoverflow](https://stackoverflow.com/questions/tagged/trains) with the 'trains' tag.
|
||||
|
||||
For feature requests or bug reports, please use [GitHub issues](https://github.com/allegroai/trains/issues).
|
||||
|
||||
Additionally, you can always find us at support@allegro.ai.
|
||||
|
||||
## Contributing
|
||||
|
||||
See the TRAINS [Guidelines for Contributing](https://github.com/allegroai/trains/blob/master/docs/contributing.md).
|
||||
|
||||
## FAQ
|
||||
|
||||
See the TRAINS [FAQ](https://github.com/allegroai/trains/blob/master/docs/faq.md).
|
||||
|
||||
<p style="font-size:0.9rem; font-weight:700; font-style:italic">May the force (and the goddess of learning rates) be with you!</p>
|
||||
_May the force (and the goddess of learning rates) be with you!_
|
||||
|
||||
|
66
docs/brief.md
Normal file
66
docs/brief.md
Normal file
@ -0,0 +1,66 @@
|
||||
# What is TRAINS?
|
||||
Behind every great scientist are great repeatable methods. Sadly, this is easier said than done.
|
||||
|
||||
When talented scientists, engineers, or developers work on their own, a mess may be unavoidable.
|
||||
Yet, it may still be manageable. However, with time and more people joining your project, managing the clutter takes
|
||||
its toll on productivity. As your project moves toward production, visibility and provenance for scaling your
|
||||
deep-learning efforts are a must.
|
||||
|
||||
For teams or entire companies, TRAINS logs everything in one central server and takes on the responsibilities for
|
||||
visibility and provenance so productivity does not suffer. TRAINS records and manages various deep learning
|
||||
research workloads and does so with practically zero integration costs.
|
||||
|
||||
We designed TRAINS specifically to require effortless integration so that teams can preserve their existing methods
|
||||
and practices. Use it on a daily basis to boost collaboration and visibility, or use it to automatically collect
|
||||
your experimentation logs, outputs, and data to one centralized server.
|
||||
|
||||
## Main Features
|
||||
|
||||
* Integrate with your current work flow with minimal effort
|
||||
* Seamless integration with leading frameworks, including: *PyTorch*, *TensorFlow*, *Keras*, and others coming soon
|
||||
* Support for *Jupyter Notebook* (see [trains-jupyter-plugin](https://github.com/allegroai/trains-jupyter-plugin))
|
||||
and *PyCharm* remote debugging (see [trains-pycharm-plugin](https://github.com/allegroai/trains-pycharm-plugin))
|
||||
* Log everything. Experiments become truly repeatable
|
||||
* Model logging with **automatic association** of **model + code + parameters + initial weights**
|
||||
* Automatically create a copy of models on centralized storage
|
||||
([supports shared folders, S3, GS,](https://github.com/allegroai/trains/blob/master/docs/faq.md#i-read-there-is-a-feature-for-centralized-model-storage-how-do-i-use-it-) and Azure is coming soon!)
|
||||
* Share and collaborate
|
||||
* Multi-user process tracking and collaboration
|
||||
* Centralized server for aggregating logs, records, and general bookkeeping
|
||||
* Increase productivity
|
||||
* Comprehensive **experiment comparison**: code commits, initial weights, hyper-parameters and metric results
|
||||
* Order & Organization
|
||||
* Manage and organize your experiments in projects
|
||||
* Query capabilities; sort and filter experiments by results metrics
|
||||
* And more
|
||||
* Stop an experiment on a remote machine using the web-app
|
||||
* A field-tested, feature-rich SDK for your on-the-fly customization needs
|
||||
|
||||
## TRAINS Automatically Logs
|
||||
|
||||
* Git repository, branch, commit id and entry point (git diff coming soon)
|
||||
* Hyper-parameters, including
|
||||
* ArgParser for command line parameters with currently used values
|
||||
* Tensorflow Defines (absl-py)
|
||||
* Explicit parameters dictionary
|
||||
* Initial model weights file
|
||||
* Model snapshots
|
||||
* stdout and stderr
|
||||
* Tensorboard/TensorboardX scalars, metrics, histograms, images (with audio coming soon)
|
||||
* Matplotlib
|
||||
|
||||
## How TRAINS Works
|
||||
|
||||
TRAINS is a two part solution:
|
||||
|
||||
1. TRAINS [python package](https://pypi.org/project/trains/) (auto-magically connects your code, see [Using TRAINS](https://github.com/allegroai/trains#using-trains))
|
||||
2. [TRAINS-server](https://github.com/allegroai/trains-server) for logging, querying, control and UI ([Web-App](https://github.com/allegroai/trains-web))
|
||||
|
||||
The following diagram illustrates the interaction of the [TRAINS-server](https://github.com/allegroai/trains-server)
|
||||
and a GPU training machine using the TRAINS python package
|
||||
|
||||
<!---
|
||||

|
||||
-->
|
||||
<img src="https://github.com/allegroai/trains/blob/master/docs/system_diagram.png?raw=true" width="50%">
|
||||
|
291
docs/faq.md
291
docs/faq.md
@ -1,29 +1,69 @@
|
||||
# TRAINS FAQ
|
||||
|
||||
* [How to change the location of TRAINS configuration file](#change-config-path)
|
||||
* [How to override TRAINS credentials from OS environment](#credentials-os-env)
|
||||
* [How to sort models by a certain metric?](#custom-columns)
|
||||
General Information
|
||||
|
||||
* [How do I know a new version came out?](#new-version-auto-update)
|
||||
|
||||
Configuration
|
||||
|
||||
* [How can I change the location of TRAINS configuration file?](#change-config-path)
|
||||
* [How can I override TRAINS credentials from the OS environment?](#credentials-os-env)
|
||||
|
||||
Models
|
||||
|
||||
* [How can I sort models by a certain metric?](#custom-columns)
|
||||
* [Can I store more information on the models?](#store-more-model-info)
|
||||
* [Can I store the model configuration file as well?](#store-model-configuration)
|
||||
* [I want to add more graphs, not just with Tensorboard. Is this supported?](#more-graph-types)
|
||||
* [Is there a way to create a graph comparing hyper-parameters vs model accuracy?](#compare-graph-parameters)
|
||||
* [I noticed that all of my experiments appear as `Training`. Are there other options?](#other-experiment-types)
|
||||
* [I noticed I keep getting the message `warning: uncommitted code`. What does it mean?](#uncommitted-code-warning)
|
||||
* [Is there something TRAINS can do about uncommitted code running?](#help-uncommitted-code)
|
||||
* [I read there is a feature for centralized model storage. How do I use it?](#centralized-model-storage)
|
||||
* [I am training multiple models at the same time, but I only see one of them. What happened?](#only-last-model-appears)
|
||||
* [Can I log input and output models manually?](#manually-log-models)
|
||||
* [I am using Jupyter Notebook. Is this supported?](#jupyter-notebook)
|
||||
|
||||
Experiments
|
||||
|
||||
* [I noticed I keep getting the message `warning: uncommitted code`. What does it mean?](#uncommitted-code-warning)
|
||||
* [I do not use Argarser for hyper-parameters. Do you have a solution?](#dont-want-argparser)
|
||||
* [Git is not well supported in Jupyter, so we just gave up on committing our code. Do you have a solution?](#commit-git-in-jupyter)
|
||||
* [Can I use TRAINS with scikit-learn?](#use-scikit-learn)
|
||||
* [When using PyCharm to remotely debug a machine, the git repo is not detected. Do you have a solution?](#pycharm-remote-debug-detect-git)
|
||||
* [How do I know a new version came out?](#new-version-auto-update)
|
||||
* [I noticed that all of my experiments appear as `Training`. Are there other options?](#other-experiment-types)
|
||||
* [Sometimes I see experiments as running when in fact they are not. What's going on?](#experiment-running-but-stopped)
|
||||
* [My code throws an exception, but my experiment status is not "Failed". What happened?](#exception-not-failed)
|
||||
* [When I run my experiment, I get an SSL Connection error [CERTIFICATE_VERIFY_FAILED]. Do you have a solution?](#ssl-connection-error)
|
||||
|
||||
Graphs and Logs
|
||||
|
||||
* [The first log lines are missing from the experiment log tab. Where did they go?](#first-log-lines-missing)
|
||||
* [Can I create a graph comparing hyper-parameters vs model accuracy?](#compare-graph-parameters)
|
||||
* [I want to add more graphs, not just with Tensorboard. Is this supported?](#more-graph-types)
|
||||
|
||||
GIT and Storage
|
||||
|
||||
* [Is there something TRAINS can do about uncommitted code running?](#help-uncommitted-code)
|
||||
* [I read there is a feature for centralized model storage. How do I use it?](#centralized-model-storage)
|
||||
* [When using PyCharm to remotely debug a machine, the git repo is not detected. Do you have a solution?](#pycharm-remote-debug-detect-git)
|
||||
* [Git is not well supported in Jupyter, so we just gave up on committing our code. Do you have a solution?](#commit-git-in-jupyter)
|
||||
|
||||
Jupyter and scikit-learn
|
||||
|
||||
* [I am using Jupyter Notebook. Is this supported?](#jupyter-notebook)
|
||||
* [Can I use TRAINS with scikit-learn?](#use-scikit-learn)
|
||||
* Also see, [Git and Jupyter](#commit-git-in-jupyter)
|
||||
|
||||
## General Information
|
||||
|
||||
### How do I know a new version came out? <a name="new-version-auto-update"></a>
|
||||
|
||||
Starting v0.9.3 TRAINS notifies on a new version release.
|
||||
|
||||
Example, new client version available
|
||||
```bash
|
||||
TRAINS new package available: UPGRADE to vX.Y.Z is recommended!
|
||||
```
|
||||
Example, new server version available
|
||||
```bash
|
||||
TRAINS-SERVER new version available: upgrade to vX.Y is recommended!
|
||||
```
|
||||
|
||||
|
||||
## How to change the location of TRAINS configuration file? <a name="change-config-path"></a>
|
||||
## Configuration
|
||||
|
||||
### How can I change the location of TRAINS configuration file? <a name="change-config-path"></a>
|
||||
|
||||
Set "TRAINS_CONFIG_FILE" OS environment variable to override the default configuration file location.
|
||||
|
||||
@ -31,19 +71,19 @@ Set "TRAINS_CONFIG_FILE" OS environment variable to override the default configu
|
||||
export TRAINS_CONFIG_FILE="/home/user/mytrains.conf"
|
||||
```
|
||||
|
||||
|
||||
## How to override TRAINS credentials from OS environment? <a name="credentials-os-env"></a>
|
||||
### How can I override TRAINS credentials from the OS environment? <a name="credentials-os-env"></a>
|
||||
|
||||
Set the OS environment variables below, in order to override the configuration file / defaults.
|
||||
|
||||
```bash
|
||||
export TRAINS_API_ACCESS_KEY="key_here"
|
||||
export TRAINS_API_SECRET_KEY="secret_here"
|
||||
export TRAINS_API_HOST="http://localhost:8080"
|
||||
export TRAINS_API_HOST="http://localhost:8008"
|
||||
```
|
||||
|
||||
## Models
|
||||
|
||||
## How to sort models by a certain metric? <a name="custom-columns"></a>
|
||||
### How can I sort models by a certain metric? <a name="custom-columns"></a>
|
||||
|
||||
Models are associated with the experiments that created them.
|
||||
In order to sort experiments by a specific metric, add a custom column in the experiments table,
|
||||
@ -51,8 +91,7 @@ In order to sort experiments by a specific metric, add a custom column in the ex
|
||||
<img src="https://github.com/allegroai/trains/blob/master/docs/screenshots/set_custom_column.png?raw=true" width=25%>
|
||||
<img src="https://github.com/allegroai/trains/blob/master/docs/screenshots/custom_column.png?raw=true" width=25%>
|
||||
|
||||
|
||||
## Can I store more information on the models? <a name="store-more-model-info"></a>
|
||||
### Can I store more information on the models? <a name="store-more-model-info"></a>
|
||||
|
||||
#### For example, can I store enumeration of classes?
|
||||
|
||||
@ -62,7 +101,7 @@ Yes! Use the `Task.set_model_label_enumeration()` method:
|
||||
Task.current_task().set_model_label_enumeration( {"label": int(0), } )
|
||||
```
|
||||
|
||||
## Can I store the model configuration file as well? <a name="store-model-configuration"></a>
|
||||
### Can I store the model configuration file as well? <a name="store-model-configuration"></a>
|
||||
|
||||
Yes! Use the `Task.set_model_design()` method:
|
||||
|
||||
@ -70,34 +109,101 @@ Yes! Use the `Task.set_model_design()` method:
|
||||
Task.current_task().set_model_design("a very long text with the configuration file's content")
|
||||
```
|
||||
|
||||
## I want to add more graphs, not just with Tensorboard. Is this supported? <a name="more-graph-types"></a>
|
||||
### I am training multiple models at the same time, but I only see one of them. What happened? <a name="only-last-model-appears"></a>
|
||||
|
||||
Yes! Use a [Logger](https://github.com/allegroai/trains/blob/master/trains/logger.py) object. An instance can be always be retrieved using the `Task.current_task().get_logger()` method:
|
||||
All models can be found under the project's **Models** tab,
|
||||
that said, currently in the Experiment's information panel TRAINS shows only the last associated model.
|
||||
|
||||
This will be fixed in a future version.
|
||||
|
||||
### Can I log input and output models manually? <a name="manually-log-models"></a>
|
||||
|
||||
Yes! For example:
|
||||
|
||||
```python
|
||||
# Get a logger object
|
||||
logger = Task.current_task().get_logger()
|
||||
input_model = InputModel.import_model(link_to_initial_model_file)
|
||||
Task.current_task().connect(input_model)
|
||||
|
||||
# Report some scalar
|
||||
logger.report_scalar("loss", "classification", iteration=42, value=1.337)
|
||||
OutputModel(Task.current_task()).update_weights(link_to_new_model_file_here)
|
||||
```
|
||||
|
||||
#### **TRAINS supports:**
|
||||
* Scalars
|
||||
* Plots
|
||||
* 2D/3D Scatter Diagrams
|
||||
* Histograms
|
||||
* Surface Diagrams
|
||||
* Confusion Matrices
|
||||
* Images
|
||||
* Text logs
|
||||
See [InputModel](https://github.com/allegroai/trains/blob/master/trains/model.py#L319) and [OutputModel](https://github.com/allegroai/trains/blob/master/trains/model.py#L539) for more information.
|
||||
|
||||
For a more detailed example, see [here](https://github.com/allegroai/trains/blob/master/examples/manual_reporting.py).
|
||||
## Experiments
|
||||
|
||||
### I noticed I keep getting the message `warning: uncommitted code`. What does it mean? <a name="uncommitted-code-warning"></a>
|
||||
|
||||
TRAINS not only detects your current repository and git commit,
|
||||
but also warns you if you are using uncommitted code. TRAINS does this
|
||||
because uncommitted code means this experiment will be difficult to reproduce.
|
||||
|
||||
If you still don't care, just ignore this message - it is merely a warning.
|
||||
|
||||
### I do not use Argarser for hyper-parameters. Do you have a solution? <a name="dont-want-argparser"></a>
|
||||
|
||||
Yes! TRAINS supports using a Python dictionary for hyper-parameter logging. Just use:
|
||||
|
||||
```python
|
||||
parameters_dict = Task.current_task().connect(parameters_dict)
|
||||
```
|
||||
|
||||
From this point onward, not only are the dictionary key/value pairs stored as part of the experiment, but any changes to the dictionary will be automatically updated in the task's information.
|
||||
|
||||
|
||||
## Is there a way to create a graph comparing hyper-parameters vs model accuracy? <a name="compare-graph-parameters"></a>
|
||||
### I noticed that all of my experiments appear as `Training`. Are there other options? <a name="other-experiment-types"></a>
|
||||
|
||||
Yes, You can manually create a plot with a single point X-axis for the hyper-parameter value,
|
||||
Yes! When creating experiments and calling `Task.init`, you can provide an experiment type.
|
||||
The currently supported types are `Task.TaskTypes.training` and `Task.TaskTypes.testing`. For example:
|
||||
|
||||
```python
|
||||
task = Task.init(project_name, task_name, Task.TaskTypes.testing)
|
||||
```
|
||||
|
||||
If you feel we should add a few more, let us know in the [issues](https://github.com/allegroai/trains/issues) section.
|
||||
|
||||
### Sometimes I see experiments as running when in fact they are not. What's going on? <a name="experiment-running-but-stopped"></a>
|
||||
|
||||
TRAINS monitors your Python process. When the process exits in an orderly fashion, TRAINS closes the experiment.
|
||||
|
||||
When the process crashes and terminates abnormally, the stop signal is sometimes missed. In such a case, you can safely right click the experiment in the Web-App and stop it.
|
||||
|
||||
## My code throws an exception, but my experiment status is not "Failed". What happened? <a name="exception-not-failed"></a>
|
||||
|
||||
This issue was resolved in v0.9.2. Upgrade TRAINS:
|
||||
|
||||
```pip install -U trains```
|
||||
|
||||
## When I run my experiment, I get an SSL Connection error [CERTIFICATE_VERIFY_FAILED]. Do you have a solution? <a name="ssl-connection-error"></a>
|
||||
|
||||
Your firewall may be preventing the connection. Try one of the following solutons:
|
||||
|
||||
* Direct python "requests" to use the enterprise certificate file by setting the OS environment variables CURL_CA_BUNDLE or REQUESTS_CA_BUNDLE.
|
||||
|
||||
You can see a detailed discussion at [https://stackoverflow.com/questions/48391750/disable-python-requests-ssl-validation-for-an-imported-module](https://stackoverflow.com/questions/48391750/disable-python-requests-ssl-validation-for-an-imported-module).
|
||||
|
||||
2. Disable certificate verification (for security reasons, this is not recommended):
|
||||
|
||||
1. Upgrade TRAINS to the current version:
|
||||
|
||||
```pip install -U trains```
|
||||
|
||||
1. Create a new **trains.conf** configuration file (sample file [here](https://github.com/allegroai/trains/blob/master/docs/trains.conf)), containing:
|
||||
|
||||
```api { verify_certificate = False }```
|
||||
|
||||
1. Copy the new **trains.conf** file to ~/trains.conf (on Windows: C:\Users\your_username\trains.conf)
|
||||
|
||||
## Graphs and Logs
|
||||
|
||||
### The first log lines are missing from the experiment log tab. Where did they go? <a name="first-log-lines-missing"></a>
|
||||
|
||||
Due to speed/optimization issues, we opted to display only the last several hundred log lines.
|
||||
|
||||
You can always downloaded the full log as a file using the Web-App.
|
||||
|
||||
### Can I create a graph comparing hyper-parameters vs model accuracy? <a name="compare-graph-parameters"></a>
|
||||
|
||||
Yes, you can manually create a plot with a single point X-axis for the hyper-parameter value,
|
||||
and Y-Axis for the accuracy. For example:
|
||||
|
||||
```python
|
||||
@ -123,34 +229,40 @@ Task.current_task().get_logger().report_vector(
|
||||
|
||||
<img src="https://github.com/allegroai/trains/blob/master/docs/screenshots/compare_plots_hist.png?raw=true" width="50%">
|
||||
|
||||
## I noticed that all of my experiments appear as `Training`. Are there other options? <a name="other-experiment-types"></a>
|
||||
### I want to add more graphs, not just with Tensorboard. Is this supported? <a name="more-graph-types"></a>
|
||||
|
||||
Yes! When creating experiments and calling `Task.init`, you can provide an experiment type.
|
||||
The currently supported types are `Task.TaskTypes.training` and `Task.TaskTypes.testing`. For example:
|
||||
Yes! Use a [Logger](https://github.com/allegroai/trains/blob/master/trains/logger.py) object. An instance can be always be retrieved using the `Task.current_task().get_logger()` method:
|
||||
|
||||
```python
|
||||
task = Task.init(project_name, task_name, Task.TaskTypes.testing)
|
||||
# Get a logger object
|
||||
logger = Task.current_task().get_logger()
|
||||
|
||||
# Report some scalar
|
||||
logger.report_scalar("loss", "classification", iteration=42, value=1.337)
|
||||
```
|
||||
|
||||
If you feel we should add a few more, let us know in the [issues](https://github.com/allegroai/trains/issues) section.
|
||||
#### **TRAINS supports:**
|
||||
|
||||
* Scalars
|
||||
* Plots
|
||||
* 2D/3D Scatter Diagrams
|
||||
* Histograms
|
||||
* Surface Diagrams
|
||||
* Confusion Matrices
|
||||
* Images
|
||||
* Text logs
|
||||
|
||||
## I noticed I keep getting the message `warning: uncommitted code`. What does it mean? <a name="uncommitted-code-warning"></a>
|
||||
For a more detailed example, see [here](https://github.com/allegroai/trains/blob/master/examples/manual_reporting.py).
|
||||
|
||||
TRAINS not only detects your current repository and git commit,
|
||||
but also warns you if you are using uncommitted code. TRAINS does this
|
||||
because uncommitted code means this experiment will be difficult to reproduce.
|
||||
## Git and Storage
|
||||
|
||||
If you still don't care, just ignore this message - it is merely a warning.
|
||||
|
||||
|
||||
## Is there something TRAINS can do about uncommitted code running? <a name="help-uncommitted-code"></a>
|
||||
### Is there something TRAINS can do about uncommitted code running? <a name="help-uncommitted-code"></a>
|
||||
|
||||
Yes! TRAINS currently stores the git diff as part of the experiment's information.
|
||||
The Web-App will soon present the git diff as well. This is coming very soon!
|
||||
|
||||
|
||||
## I read there is a feature for centralized model storage. How do I use it? <a name="centralized-model-storage"></a>
|
||||
### I read there is a feature for centralized model storage. How do I use it? <a name="centralized-model-storage"></a>
|
||||
|
||||
When calling `Task.init()`, providing the `output_uri` parameter allows you to specify the location in which model snapshots will be stored.
|
||||
|
||||
@ -182,74 +294,25 @@ taks = Task.init(project_name, task_name, output_uri="gs://bucket-name/folder")
|
||||
For a more detailed example, see [here](https://github.com/allegroai/trains/blob/master/docs/trains.conf#L55).
|
||||
|
||||
|
||||
## I am training multiple models at the same time, but I only see one of them. What happened? <a name="only-last-model-appears"></a>
|
||||
|
||||
Although all models can be found under the project's **Models** tab, TRAINS currently shows only the last model associated with an experiment in the experiment's information panel.
|
||||
### When using PyCharm to remotely debug a machine, the git repo is not detected. Do you have a solution? <a name="pycharm-remote-debug-detect-git"></a>
|
||||
|
||||
This will be fixed in a future version.
|
||||
Yes! Since this is such a common occurrence, we created a PyCharm plugin that allows a remote debugger to grab your local repository / commit ID. See our [TRAINS PyCharm Plugin](https://github.com/allegroai/trains-pycharm-plugin) repository for instructions and [latest release](https://github.com/allegroai/trains-pycharm-plugin/releases).
|
||||
|
||||
## Can I log input and output models manually? <a name="manually-log-models"></a>
|
||||
|
||||
Yes! For example:
|
||||
|
||||
```python
|
||||
input_model = InputModel.import_model(link_to_initial_model_file)
|
||||
Task.current_task().connect(input_model)
|
||||
|
||||
OutputModel(Task.current_task()).update_weights(link_to_new_model_file_here)
|
||||
```
|
||||
|
||||
See [InputModel](https://github.com/allegroai/trains/blob/master/trains/model.py#L319) and [OutputModel](https://github.com/allegroai/trains/blob/master/trains/model.py#L539) for more information.
|
||||
|
||||
|
||||
## I am using Jupyter Notebook. Is this supported? <a name="jupyter-notebook"></a>
|
||||
|
||||
Yes! Jupyter Notebook is supported. See [TRAINS Jupyter Plugin](https://github.com/allegroai/trains-jupyter-plugin).
|
||||
|
||||
|
||||
## I do not use Argarser for hyper-parameters. Do you have a solution? <a name="dont-want-argparser"></a>
|
||||
|
||||
Yes! TRAINS supports using a Python dictionary for hyper-parameter logging. Just call:
|
||||
|
||||
```python
|
||||
parameters_dict = Task.current_task().connect(parameters_dict)
|
||||
```
|
||||
|
||||
From this point onward, not only are the dictionary key/value pairs stored as part of the experiment, but any changes to the dictionary will be automatically updated in the task's information.
|
||||
|
||||
|
||||
## Git is not well supported in Jupyter, so we just gave up on committing our code. Do you have a solution? <a name="commit-git-in-jupyter"></a>
|
||||
### Git is not well supported in Jupyter, so we just gave up on committing our code. Do you have a solution? <a name="commit-git-in-jupyter"></a>
|
||||
|
||||
Yes! Check our [TRAINS Jupyter Plugin](https://github.com/allegroai/trains-jupyter-plugin). This plugin allows you to commit your notebook directly from Jupyter. It also saves the Python version of your code and creates an updated `requirements.txt` so you know which packages you were using.
|
||||
|
||||
|
||||
## Can I use TRAINS with scikit-learn? <a name="use-scikit-learn"></a>
|
||||
## Jupyter and scikit-learn
|
||||
|
||||
### I am using Jupyter Notebook. Is this supported? <a name="jupyter-notebook"></a>
|
||||
|
||||
Yes! Jupyter Notebook is supported. See [TRAINS Jupyter Plugin](https://github.com/allegroai/trains-jupyter-plugin).
|
||||
|
||||
|
||||
### Can I use TRAINS with scikit-learn? <a name="use-scikit-learn"></a>
|
||||
|
||||
Yes! `scikit-learn` is supported. Everything you do is logged.
|
||||
|
||||
**NOTE**: Models are not automatically logged because in most cases, scikit-learn will simply pickle the object to files so there is no underlying frame we can connect to.
|
||||
|
||||
|
||||
## When using PyCharm to remotely debug a machine, the git repo is not detected. Do you have a solution? <a name="pycharm-remote-debug-detect-git"></a>
|
||||
|
||||
Yes! Since this is such a common occurrence, we created a PyCharm plugin that allows a remote debugger to grab your local repository / commit ID. See our [TRAINS PyCharm Plugin](https://github.com/allegroai/trains-pycharm-plugin) repository for instructions and [latest release](https://github.com/allegroai/trains-pycharm-plugin/releases).
|
||||
|
||||
|
||||
## How do I know a new version came out? <a name="new-version-auto-update"></a>
|
||||
|
||||
TRAINS does not yet support auto-update checks. We hope to add this feature soon.
|
||||
|
||||
|
||||
## Sometimes I see experiments as running when in fact they are not. What's going on? <a name="experiment-running-but-stopped"></a>
|
||||
|
||||
TRAINS monitors your Python process. When the process exits in an orderly fashion, TRAINS closes the experiment.
|
||||
|
||||
When the process crashes and terminates abnormally, the stop signal is sometimes missed. In such a case, you can safely right click the experiment in the Web-App and stop it.
|
||||
|
||||
|
||||
## The first log lines are missing from the experiment log tab. Where did they go? <a name="first-log-lines-missing"></a>
|
||||
|
||||
Due to speed/optimization issues, we opted to display only the last several hundred log lines.
|
||||
|
||||
You can always downloaded the full log as a file using the Web-App.
|
||||
|
||||
|
Binary file not shown.
Before Width: | Height: | Size: 1.6 MiB After Width: | Height: | Size: 1.6 MiB |
Loading…
Reference in New Issue
Block a user