mirror of
https://github.com/clearml/clearml-docs
synced 2025-06-26 18:17:44 +00:00
Small edits (#588)
This commit is contained in:
parent
53ebbde06d
commit
cd238c746f
@ -87,7 +87,7 @@ clearml-agent daemon [-h] [--foreground] [--queue QUEUES [QUEUES ...]] [--order-
|
||||
|`--create-queue`| If the queue name provided with `--queue` does not exist in the server, create it on-the-fly and use it.|<img src="/docs/latest/icons/ico-optional-yes.svg" alt="Yes" className="icon size-md center-md" />|
|
||||
|`--detached`| Run agent in the background. The `clearml-agent` command returns immediately.|<img src="/docs/latest/icons/ico-optional-yes.svg" alt="Yes" className="icon size-md center-md" />|
|
||||
|`--docker`| Run in Docker mode. Execute the Task inside a Docker container. To specify the image name and optional arguments, either: <ul><li> Use `--docker <image_name> <args>` on the command line, or </li><li>Use `--docker` on the command line, and specify the default image name and arguments in the configuration file.</li></ul> Environment variable settings for Docker containers: <ul><li>`CLEARML_DOCKER_SKIP_GPUS_FLAG` - Ignore the `--gpus` flag inside the Docker container. This also lets you execute ClearML Agent using Docker versions earlier than 19.03.</li><li>`NVIDIA_VISIBLE_DEVICES` - Limit GPU visibility for the Docker container.</li><li> `CLEARML_AGENT_GIT_USER` and `CLEARML_AGENT_GIT_PASS` - Pass these credentials to the Docker container at execution.</li></ul>|<img src="/docs/latest/icons/ico-optional-yes.svg" alt="Yes" className="icon size-md center-md" />|
|
||||
|`--downtime`| Specify downtime for clearml-agent in `<hours> <days>` format. For example, use `09-13 TUE` to set Tuesday's downtime to 09-13. <br/><br/>NOTE: <ul><li>This feature is available under the ClearML Enterprise plan</li><li>Make sure to have only one of uptime / downtime configuration and not both.</li></ul> |<img src="/docs/latest/icons/ico-optional-yes.svg" alt="Yes" className="icon size-md center-md" />|
|
||||
|`--downtime`| Specify downtime for clearml-agent in `<hours> <days>` format. For example, use `09-13 TUE` to set Tuesday's downtime to 09-13. <br/><br/>NOTES: <ul><li>This feature is available under the ClearML Enterprise plan</li><li>Make sure to configure only `--uptime` or `--downtime`, but not both.</li></ul> |<img src="/docs/latest/icons/ico-optional-yes.svg" alt="Yes" className="icon size-md center-md" />|
|
||||
|`--dynamic-gpus`| Allow to dynamically allocate GPUs based on queue properties, configure with `--queue <queue_name>=<num_gpus>`. For example: `--dynamic-gpus --queue dual_gpus=2 single_gpu=1` <br/><br/>NOTE: This feature is available under the ClearML Enterprise plan|<img src="/docs/latest/icons/ico-optional-yes.svg" alt="Yes" className="icon size-md center-md" />|
|
||||
|`--force-current-version`| To use your current version of ClearML Agent when running in Docker mode (the `--docker` argument is specified), instead of the latest ClearML Agent version which is automatically installed, specify `force-current-version`. <br/><br/> For example, if your current ClearML Agent version is `0.13.1`, but the latest version of ClearML Agent is `0.13.3`, use `--force-current-version` and your Task will execute in the Docker container with ClearML Agent version `0.13.1`.|<img src="/docs/latest/icons/ico-optional-yes.svg" alt="Yes" className="icon size-md center-md" />|
|
||||
|`--foreground`| Pipe full log to stdout/stderr. Do not use this option if running in background.|<img src="/docs/latest/icons/ico-optional-yes.svg" alt="Yes" className="icon size-md center-md" />|
|
||||
@ -103,7 +103,7 @@ clearml-agent daemon [-h] [--foreground] [--queue QUEUES [QUEUES ...]] [--order-
|
||||
|`--standalone-mode`| Do not use any network connects. This assumes all requirements are pre-installed.|<img src="/docs/latest/icons/ico-optional-yes.svg" alt="Yes" className="icon size-md center-md" />|
|
||||
|`--status`| Print the worker's schedule (uptime properties, server's runtime properties and listening queues)|<img src="/docs/latest/icons/ico-optional-yes.svg" alt="Yes" className="icon size-md center-md" />|
|
||||
|`--stop`| Terminate a running ClearML Agent, if other arguments are the same. If no additional arguments are provided, agents are terminated in lexicographical order.|<img src="/docs/latest/icons/ico-optional-yes.svg" alt="Yes" className="icon size-md center-md" />|
|
||||
|`--uptime`| Specify uptime for clearml-agent in `<hours> <days>` format. For example, use `17-20 TUE` to set Tuesday's uptime to 17-20. <br/><br/>NOTES<ul><li>This feature is available under the ClearML Enterprise plan </li><li>Make sure to have only one of uptime / downtime configuration and not both.</li></ul>|<img src="/docs/latest/icons/ico-optional-yes.svg" alt="Yes" className="icon size-md center-md" />|
|
||||
|`--uptime`| Specify uptime for clearml-agent in `<hours> <days>` format. For example, use `17-20 TUE` to set Tuesday's uptime to 17-20. <br/><br/>NOTES:<ul><li>This feature is available under the ClearML Enterprise plan </li><li>Make sure to configure only `--uptime` or `--downtime`, but not both.</li></ul>|<img src="/docs/latest/icons/ico-optional-yes.svg" alt="Yes" className="icon size-md center-md" />|
|
||||
|`--use-owner-token`| Generate and use the task owner's token for the execution of the task.|<img src="/docs/latest/icons/ico-optional-yes.svg" alt="Yes" className="icon size-md center-md" />|
|
||||
|
||||
## execute
|
||||
|
@ -627,7 +627,7 @@ Notice that if one of the frameworks loads an existing weights file, the running
|
||||
"Input Model", pointing directly to the original training task's model. This makes it easy to get the full lineage of
|
||||
every trained and used model in our system!
|
||||
|
||||
Models loaded by the ML framework appear under the "Input Models" section, under the Artifacts tab in the ClearML UI.
|
||||
Models loaded by the ML framework appear in an experiment's **Artifacts** tab under the "Input Models" section in the ClearML UI.
|
||||
|
||||
### Setting Upload Destination
|
||||
|
||||
|
@ -104,7 +104,7 @@ At the beginning of your code, import the `clearml` package:
|
||||
from clearml import Task
|
||||
```
|
||||
|
||||
:::note Full Automatic Logging
|
||||
:::tip Full Automatic Logging
|
||||
To ensure full automatic logging, it is recommended to import the `clearml` package at the top of your entry script.
|
||||
:::
|
||||
|
||||
@ -128,12 +128,11 @@ ClearML results page: https://app.clear.ml/projects/4043a1657f374e9298649c6ba72a
|
||||
**That’s it!** You are done integrating ClearML with your code :)
|
||||
|
||||
Now, [command-line arguments](../../fundamentals/hyperparameters.md#tracking-hyperparameters), [console output](../../fundamentals/logger.md#types-of-logged-results) as well as Tensorboard and Matplotlib will automatically be logged in the UI under the created Task.
|
||||
<br/>
|
||||
|
||||
Sit back, relax, and watch your models converge :) or continue to see what else can be done with ClearML [here](ds_second_steps.md).
|
||||
|
||||
## YouTube Playlist
|
||||
|
||||
Or watch the YouTube Getting Started Playlist on our YouTube Channel!
|
||||
Or watch the Getting Started Playlist on our YouTube Channel!
|
||||
|
||||
[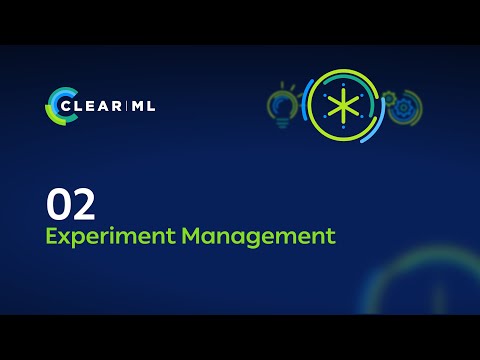](https://www.youtube.com/watch?v=bjWwZAzDxTY&list=PLMdIlCuMqSTnoC45ME5_JnsJX0zWqDdlO&index=2)
|
||||
|
@ -23,7 +23,7 @@ Once you have a Task object you can query the state of the Task, get its model,
|
||||
|
||||
## Log Hyperparameters
|
||||
|
||||
For full reproducibility, it's paramount to save Hyperparameters for each experiment. Since Hyperparameters can have substantial impact
|
||||
For full reproducibility, it's paramount to save hyperparameters for each experiment. Since hyperparameters can have substantial impact
|
||||
on Model performance, saving and comparing these between experiments is sometimes the key to understanding model behavior.
|
||||
|
||||
ClearML supports logging `argparse` module arguments out of the box, so once ClearML is integrated into the code, it automatically logs all parameters provided to the argument parser.
|
||||
@ -43,7 +43,7 @@ Check [this](../../fundamentals/hyperparameters.md) out for all Hyperparameter l
|
||||
ClearML lets you easily store the output products of an experiment - Model snapshot / weights file, a preprocessing of your data, feature representation of data and more!
|
||||
|
||||
Essentially, artifacts are files (or python objects) uploaded from a script and are stored alongside the Task.
|
||||
These Artifacts can be easily accessed by the web UI or programmatically.
|
||||
These artifacts can be easily accessed by the web UI or programmatically.
|
||||
|
||||
Artifacts can be stored anywhere, either on the ClearML server, or any object storage solution or shared folder.
|
||||
See all [storage capabilities](../../integrations/storage.md).
|
||||
@ -73,9 +73,9 @@ Check out all [artifact logging](../../clearml_sdk/task_sdk.md#artifacts) option
|
||||
|
||||
### Using Artifacts
|
||||
|
||||
Logged Artifacts can be used by other Tasks, whether it's a pre-trained Model or processed data.
|
||||
To use an Artifact, first we have to get an instance of the Task that originally created it,
|
||||
then we either download it and get its path, or get the Artifact object directly.
|
||||
Logged artifacts can be used by other Tasks, whether it's a pre-trained Model or processed data.
|
||||
To use an artifact, first we have to get an instance of the Task that originally created it,
|
||||
then we either download it and get its path, or get the artifact object directly.
|
||||
|
||||
For example, using a previously generated preprocessed data.
|
||||
|
||||
@ -84,7 +84,7 @@ preprocess_task = Task.get_task(task_id='preprocessing_task_id')
|
||||
local_csv = preprocess_task.artifacts['data'].get_local_copy()
|
||||
```
|
||||
|
||||
The `task.artifacts` is a dictionary where the keys are the Artifact names, and the returned object is the Artifact object.
|
||||
The `task.artifacts` is a dictionary where the keys are the artifact names, and the returned object is the artifact object.
|
||||
Calling `get_local_copy()` returns a local cached copy of the artifact. Therefore, next time we execute the code, we don't
|
||||
need to download the artifact again.
|
||||
Calling `get()` gets a deserialized pickled object.
|
||||
@ -130,7 +130,7 @@ Like before we have to get the instance of the Task training the original weight
|
||||
:::note
|
||||
Using TensorFlow, the snapshots are stored in a folder, meaning the `local_weights_path` will point to a folder containing your requested snapshot.
|
||||
:::
|
||||
As with Artifacts, all models are cached, meaning the next time we run this code, no model needs to be downloaded.
|
||||
As with artifacts, all models are cached, meaning the next time we run this code, no model needs to be downloaded.
|
||||
Once one of the frameworks will load the weights file, the running Task will be automatically updated with “Input Model” pointing directly to the original training Task’s Model.
|
||||
This feature lets you easily get a full genealogy of every trained and used model by your system!
|
||||
|
||||
|
@ -276,7 +276,7 @@ frame = DatasetVersion.get_single_frame(
|
||||
To access a SingleFrame, the following must be specified:
|
||||
* `frame_id`, which can be found in the WebApp, in the frame's **FRAMEGROUP DETAILS**
|
||||
* The frame's dataset - either with `dataset_name` or `dataset_id`
|
||||
* The dataset version - either with `version_id` or `version_name`
|
||||
* The dataset version - either with `version_id` or `version_name`
|
||||
|
||||
### Updating SingleFrames
|
||||
|
||||
|
@ -6,7 +6,7 @@ title: Project Dashboard
|
||||
The ClearML Project Dashboard App is available under the ClearML Pro plan
|
||||
:::
|
||||
|
||||
The Project Dashboard Application provides an overview of a project or workspace’s progress. It presents an aggregated
|
||||
The Project Dashboard Application provides an overview of a project or workspace’s progress. It presents an aggregated
|
||||
view of task status and a chosen metric over time, as well as project GPU and worker usage. It also supports alerts/warnings
|
||||
on completed/failed Tasks via Slack integration.
|
||||
|
||||
@ -27,7 +27,7 @@ of the chosen metric over time.
|
||||
* Monitored Metric - Title - Metric title to track
|
||||
* Monitored Metric - Series - Metric series (variant) to track
|
||||
* Monitored Metric - Trend - Choose whether to track the monitored metric's highest or lowest values
|
||||
* Slack Notification (optional) - Set up Slack integration for notifications of task failure. Select the
|
||||
* Slack Notification (optional) - Set up Slack integration for notifications of task failure. Select the
|
||||
`Alert on completed experiments` under `Additional options` to set up alerts for task completions.
|
||||
* API Token - Slack workspace access token
|
||||
* Channel Name - Slack channel to which task failure alerts will be posted
|
||||
|
Loading…
Reference in New Issue
Block a user