mirror of
https://github.com/clearml/clearml-docs
synced 2025-03-09 13:42:26 +00:00
Small edits (#769)
This commit is contained in:
parent
f017ca99b3
commit
b46e7471a4
@ -190,7 +190,7 @@ dataset.add_external_files(
|
||||
)
|
||||
```
|
||||
|
||||
There is an option to add a set of files based on wildcard matching of a single string or a list of wildcards, using the
|
||||
You can add a set of files based on wildcard matching of a single string or a list of wildcards using the
|
||||
`wildcard` parameter. Specify whether to match the wildcard files recursively using the `recursive` parameter.
|
||||
|
||||
```python
|
||||
|
@ -71,12 +71,16 @@ After invoking `Task.init` in a script, ClearML starts its automagical logging,
|
||||
* [TensorFlow](../integrations/tensorflow.md)
|
||||
* [Keras](../integrations/keras.md)
|
||||
* [PyTorch](../integrations/pytorch.md)
|
||||
* [scikit-learn](../integrations/scikit_learn.md)
|
||||
* [XGBoost](../integrations/xgboost.md)
|
||||
* [FastAI](../integrations/fastai.md)
|
||||
* [AutoKeras](../integrations/autokeras.md)
|
||||
* [CatBoost](../integrations/catboost.md)
|
||||
* [Fast.ai](../integrations/fastai.md)
|
||||
* [LightGBM](../integrations/lightgbm.md)
|
||||
* [MegEngine](../integrations/megengine.md)
|
||||
* [CatBoost](../integrations/catboost.md)
|
||||
* [MONAI](../integrations/monai.md)
|
||||
* [scikit-learn](../integrations/scikit_learn.md) (only using joblib)
|
||||
* [XGBoost](../integrations/xgboost.md) (only using joblib)
|
||||
* [YOLOv8](../integrations/yolov8.md)
|
||||
* [YOLOv5](../integrations/yolov5.md)
|
||||
|
||||
* **Metrics, scalars, plots, debug images** reported through supported frameworks, including:
|
||||
* [Matplotlib](../integrations/matplotlib.md)
|
||||
@ -172,8 +176,8 @@ It's possible to always create a new task by passing `reuse_last_task_id=False`.
|
||||
See full `Task.init` reference [here](../references/sdk/task.md#taskinit).
|
||||
|
||||
### Continuing Task Execution
|
||||
You can continue the execution of a previously run task using the `continue_last_task` parameter of the `Task.init`
|
||||
method. This will retain all of its previous artifacts / models / logs.
|
||||
You can continue the execution of a previously run task using the `continue_last_task` parameter of `Task.init()`.
|
||||
This will retain all of its previous artifacts / models / logs.
|
||||
|
||||
The task will continue reporting its outputs based on the iteration in which it had left off. For example: a task's last
|
||||
train/loss scalar reported was for iteration 100, when continued, the next report will be as iteration 101.
|
||||
@ -432,7 +436,7 @@ A compelling workflow is:
|
||||
1. Run code on a development machine for a few iterations, or just set up the environment.
|
||||
1. Move the execution to a beefier remote machine for the actual training.
|
||||
|
||||
Use the [`Task.execute_remotely`](../references/sdk/task.md#execute_remotely) method to implement this workflow. This method
|
||||
Use [`Task.execute_remotely()`](../references/sdk/task.md#execute_remotely) to implement this workflow. This method
|
||||
stops the current manual execution, and then re-runs it on a remote machine.
|
||||
|
||||
For example:
|
||||
|
@ -3,8 +3,8 @@ title: Best Practices
|
||||
---
|
||||
|
||||
This section talks about what made us design ClearML the way we did and how it reflects on AI workflows.
|
||||
While ClearML was designed to fit into any workflow, we do feel that working as we describe below brings a lot of advantages from organizing one's workflow
|
||||
and furthermore, preparing it to scale in the long term.
|
||||
While ClearML was designed to fit into any workflow, the practices described below brings a lot of advantages from organizing one's workflow
|
||||
to preparing it to scale in the long term.
|
||||
|
||||
:::important
|
||||
The below is only our opinion. ClearML was designed to fit into any workflow whether it conforms to our way or not!
|
||||
@ -22,7 +22,7 @@ During early stages of model development, while code is still being modified hea
|
||||
the model and ensure that you choose a model that makes sense, and the training procedure works. Can be used to provide initial models for testing.
|
||||
|
||||
The abovementioned setups might be folded into each other and that's great! If you have a GPU machine for each researcher, that's awesome!
|
||||
The goal of this phase is to get a code, dataset, and environment setup, so you can start digging to find the best model!
|
||||
The goal of this phase is to get a code, dataset, and environment set up, so you can start digging to find the best model!
|
||||
|
||||
- [ClearML SDK](../../clearml_sdk/clearml_sdk.md) should be integrated into your code (check out [Getting Started](ds_first_steps.md)).
|
||||
This helps visualizing the results and tracking progress.
|
||||
|
@ -133,6 +133,6 @@ Sit back, relax, and watch your models converge :) or continue to see what else
|
||||
|
||||
## YouTube Playlist
|
||||
|
||||
Or watch the Getting Started Playlist on ClearML's YouTube Channel!
|
||||
Or watch the **Getting Started** playlist on ClearML's YouTube Channel!
|
||||
|
||||
[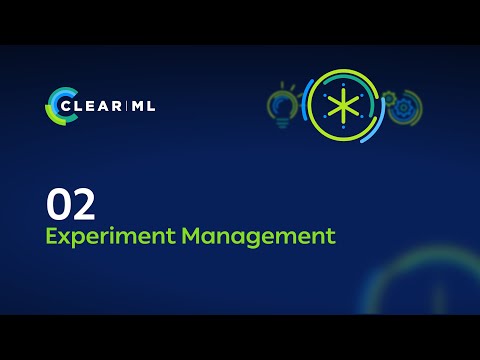](https://www.youtube.com/watch?v=bjWwZAzDxTY&list=PLMdIlCuMqSTnoC45ME5_JnsJX0zWqDdlO&index=2)
|
||||
|
@ -23,7 +23,7 @@ through parameterized data access and metadata version control.
|
||||
|
||||
Hyper-Datasets is a data management system specifically tailored for handling unstructured data, like text, audio, or
|
||||
visual data. You can create, manage, and version your datasets. Datasets can be set up to inherit from other datasets, so
|
||||
data lineages can be created, and users can track when and how their data changes. In the ClearML Enterprise's WebApp,
|
||||
data lineages can be created, and users can track when and how their data changes. In the ClearML Enterprise's [WebApp](hyperdatasets/webapp/webapp_datasets.md),
|
||||
you can view a dataset's version history, as well as its contents, including annotations, metadata, masks, and other
|
||||
information.
|
||||
|
||||
@ -32,7 +32,7 @@ information.
|
||||
The basic premise of Hyper-Datasets is that a user-formed query is a full representation of the dataset used by the ML/DL
|
||||
process. Hyper-Datasets decouple metadata from raw data files, allowing you to manipulate metadata through sophisticated
|
||||
queries and parameters that can be tracked through the experiment manager. You can clone experiments using different
|
||||
data manipulations--or **DataViews**--without changing any of the hard coded values, making these manipulations part of
|
||||
data manipulations--or [**DataViews**](hyperdatasets/dataviews.md)--without changing any of the hard coded values, making these manipulations part of
|
||||
the experiment.
|
||||
|
||||
ClearML **Enterprise**'s Hyper-Datasets supports rapid prototyping, creating new opportunities such as:
|
||||
|
@ -43,12 +43,16 @@ Automatic logging is supported for the following frameworks:
|
||||
* [TensorFlow](integrations/tensorflow.md)
|
||||
* [Keras](integrations/keras.md)
|
||||
* [PyTorch](integrations/pytorch.md)
|
||||
* [AutoKeras](integrations/autokeras.md)
|
||||
* [CatBoost](integrations/catboost.md)
|
||||
* [Fast.ai](integrations/fastai.md)
|
||||
* [LightGBM](integrations/lightgbm.md)
|
||||
* [MegEngine](integrations/megengine.md)
|
||||
* [MONAI](integrations/monai.md)
|
||||
* [scikit-learn](integrations/scikit_learn.md) (only using joblib)
|
||||
* [XGBoost](integrations/xgboost.md) (only using joblib)
|
||||
* [Fast.ai](integrations/fastai.md)
|
||||
* [MegEngine](integrations/megengine.md)
|
||||
* [CatBoost](integrations/catboost.md)
|
||||
* [MONAI](integrations/monai.md)
|
||||
* [YOLOv8](integrations/yolov8.md)
|
||||
* [YOLOv5](integrations/yolov5.md)
|
||||
|
||||
You may want more control over which models are logged. Use the `auto_connect_framework` parameter of [`Task.init()`](references/sdk/task.md#taskinit)
|
||||
to control automatic logging of frameworks.
|
||||
|
Loading…
Reference in New Issue
Block a user